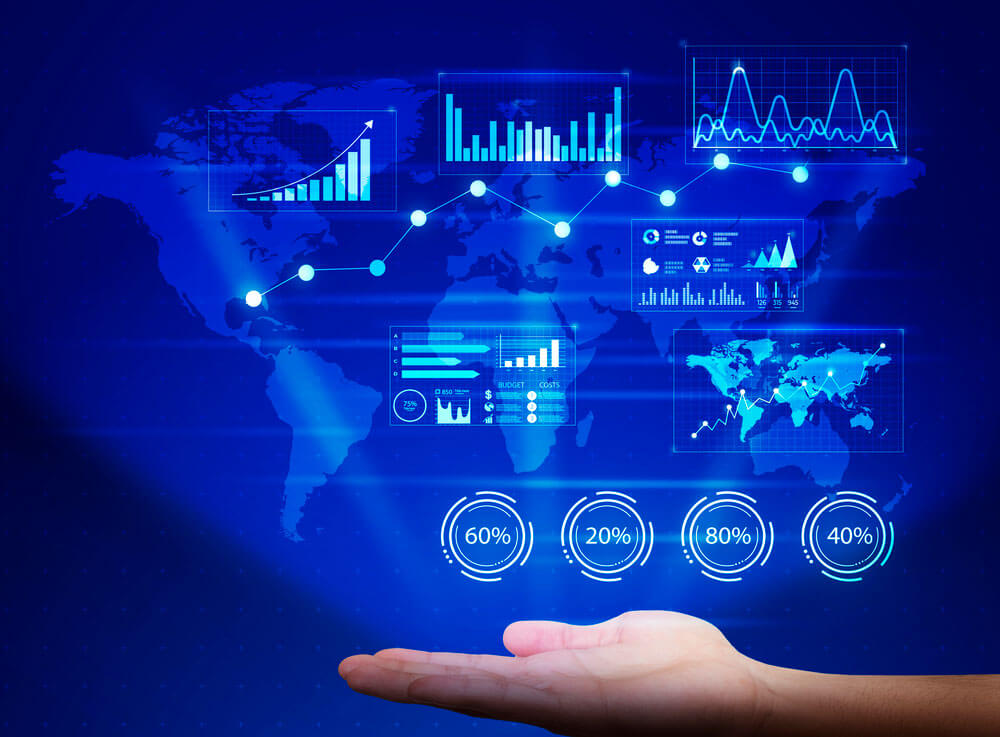
Operational Analytics - Getting Started Guide
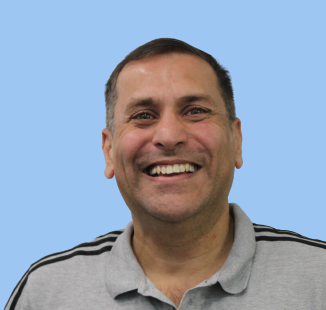
Operational Analytics is a way to bridge the last mile gap in data analytics, by making high quality, reliable data from the data warehouse available to the business teams in relevant tools of their choosing. It is a way to get the maximum out of your data, by ensuring it is not just available to select few users with access to the BI tools and reports, but also to all other members of the business teams for their day to day functioning and decision making. Typically organizations use a process called Reverse ETL to achieve this. (Learn more about Reverse ETL here) In this article we will explore the characteristics, challenges, use cases & benefits of operational analytics & how you can get started with adopting it in your organization.
Gone are the days when aggregated data insights used to sit idly in BI dashboards available to a select few, who then used these insights to create an actionable plan.
What is Operational Analytics?
With the advent of cloud computing, “data driven decision making” is not just an aspiration but a necessity for any growing business. The technology has become increasingly accessible and affordable for companies of all sizes. Modern cloud data warehouses like AWS Redshift, Google Bigquery & Snowflake with their pay as you go pricing, analytical capabilities at scale & ease of set up have made sure more and more companies are utilizing ETL tools like DataChannel, Fivetran & Stitch to create single source of truth for all their data. They then typically use BI tools like Power BI, Tableau or Looker to create dashboards & reports.
Machine Learning & AI are further used to enhance the quality of this data and the insights that can be derived from it. However, in most cases these insights and the value of these insights is limited to within the BI tools and not readily available to the wider set of functionaries in the business teams who use different tools / platforms for their day to day business activities. Imagine a case where an organization moves all their leads to a data warehouse, invest significant time and resources in building a predictive lead scoring model using machine learning, but the sales teams who are using CRM for their day to day functions never have access to the lead score as it is stuck within the data warehouse / data lake. This is typically known as the “last mile” problem in analytics.
Without bridging this gap, the true value and potential of this data can not be unlocked. Business users are then left feeling a bit like a sailor stranded at sea, surrounded by water but with nothing to drink. Typical answer to this problem is building one off ad hoc solutions to move selected data out of the warehouse after processing and into tools that the business users work in. This quickly becomes highly complex and unmanageable for the data teams and takes up most of their time and energy removing their focus from their actual tasks of studying the data and deriving insights. This is where operational analytics processes and reverse ETL tools come to the rescue.
Operational Analytics is a category of business analytics where the focus is on making the insights curated from data available to the end users who can actually put it to work in tools they use every day there by truly democratizing access to data driven decision making
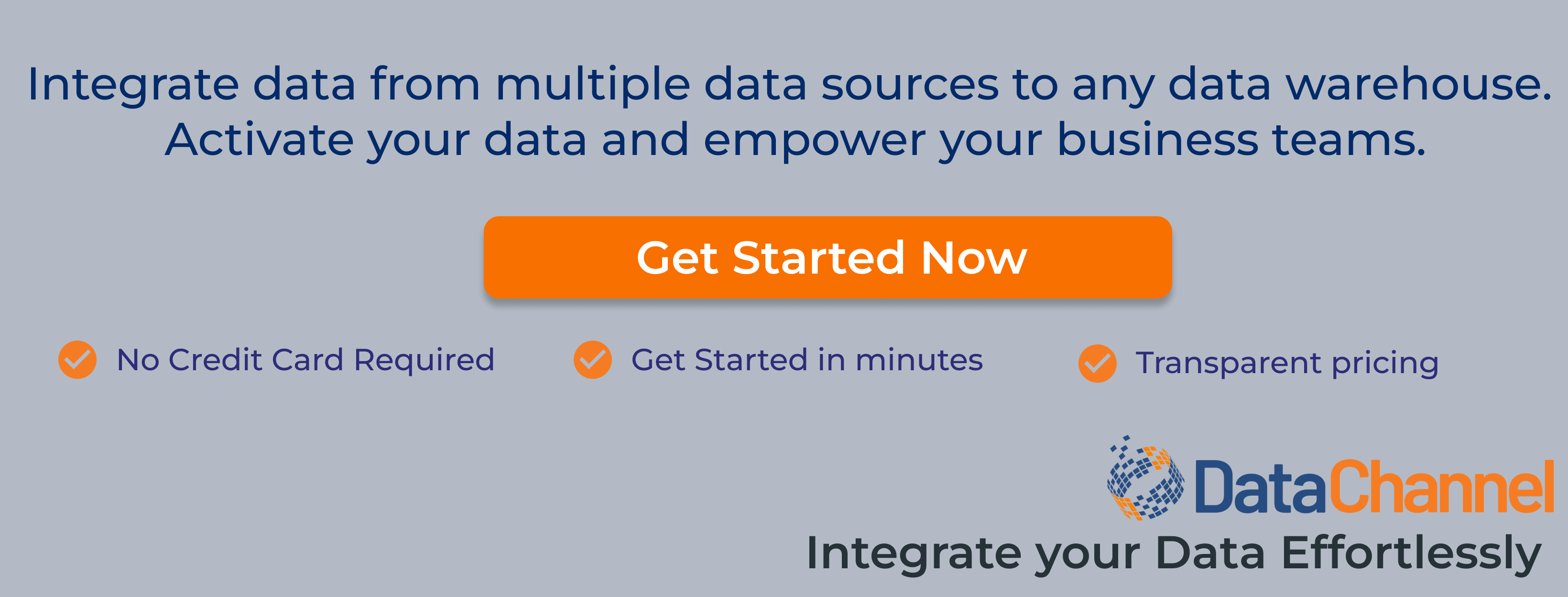
Operational Analytics - How is it different from Traditional Analytics?
While traditional analytics focuses on deriving insights from data (and presenting it in the form of dashboards and reports), operational analytics focuses on taking actions on these insights or putting them to work.
Traditional Analytics typically involves extraction, loading, transformation, cleaning and then processing of data to derive insights, uncover patterns and predict future trends from data. This is then made available through BI tools in the form of executive dashboards and periodic reports. In most cases though it is limited to monitoring a set of Key Performance Indicators (KPIs) to give insights into the state of the business process or to assist in strategic decision making.
Operational Analytics on the other hand is more focussed on taking action on the insights derived from this data in the day to day functioning and every day processes of the organization.
Let’s consider a product based SaaS company who is collecting data about its customer behavior and their product usage patterns in a data warehouse. In the traditional form of analytics this data would be used to create dashboards and reports which can be very useful for planning product strategy and taking strategic decisions. However, a sales representative when on a call with a customer, will not fall back to the BI dashboards. But if this information were available to him/her in the CRM itself, they would be able to use this to make more informed decisions and instantly impact success rates.
It is therefore evident that both these forms of analytics are not only different, but both are needed if a company truly wants to become a data driven organization and wants to extract maximum ROI from their investments into their data stack.
Operational Analytics - Where does it fit in the Modern Data Stack
The emergence of cloud data warehouses has given rise to the adoption of Modern Data Stack which typically comprises of the following layers / pieces:-
- Data Extraction & Loading using ETL Tools like DataChannel, Talend, Hevo, Fivetran etc
- Data Storage into Data Warehouses or Data Lakes
- Data Transformation using SQL, dbt or python
- BI, Dashboarding & Visualization
- Data Governance and Security Layer
While this stack gave companies a single source of truth for their data, it also quickly made them realize that now that they had access to all this high quality data, which can be utilized in the decision making and functioning of their teams. To do this, they started to create integrations to take this data out of the warehouse and into business tools like CRMs, marketing platforms, email tools etc. Quickly this started to become unmanageable and led to Reverse ETL tools like DataChannel, Census and Hightouch to start becoming a part of the data stack focussed specifically on Operational Analytics by bridging the last mile gap.
How does Operational Analytics work?
The basic premise for Operationalizing analytics is that the data warehouse is at the front and center and is treated like the single source of information for all decision making processes. Evidently, before this can happen we need to ensure that the data going into the warehouse is not only clean but also complete in all respects. A quality ETL tool and process implemented with best practices is an essential prerequisite for this. Once you have this, implementing a Reverse ETL tool like DataChannel on top of this will open up an unlimited array of opportunities for activating and operationalizing your data. Ideally a modern data stack should now look something like this:-
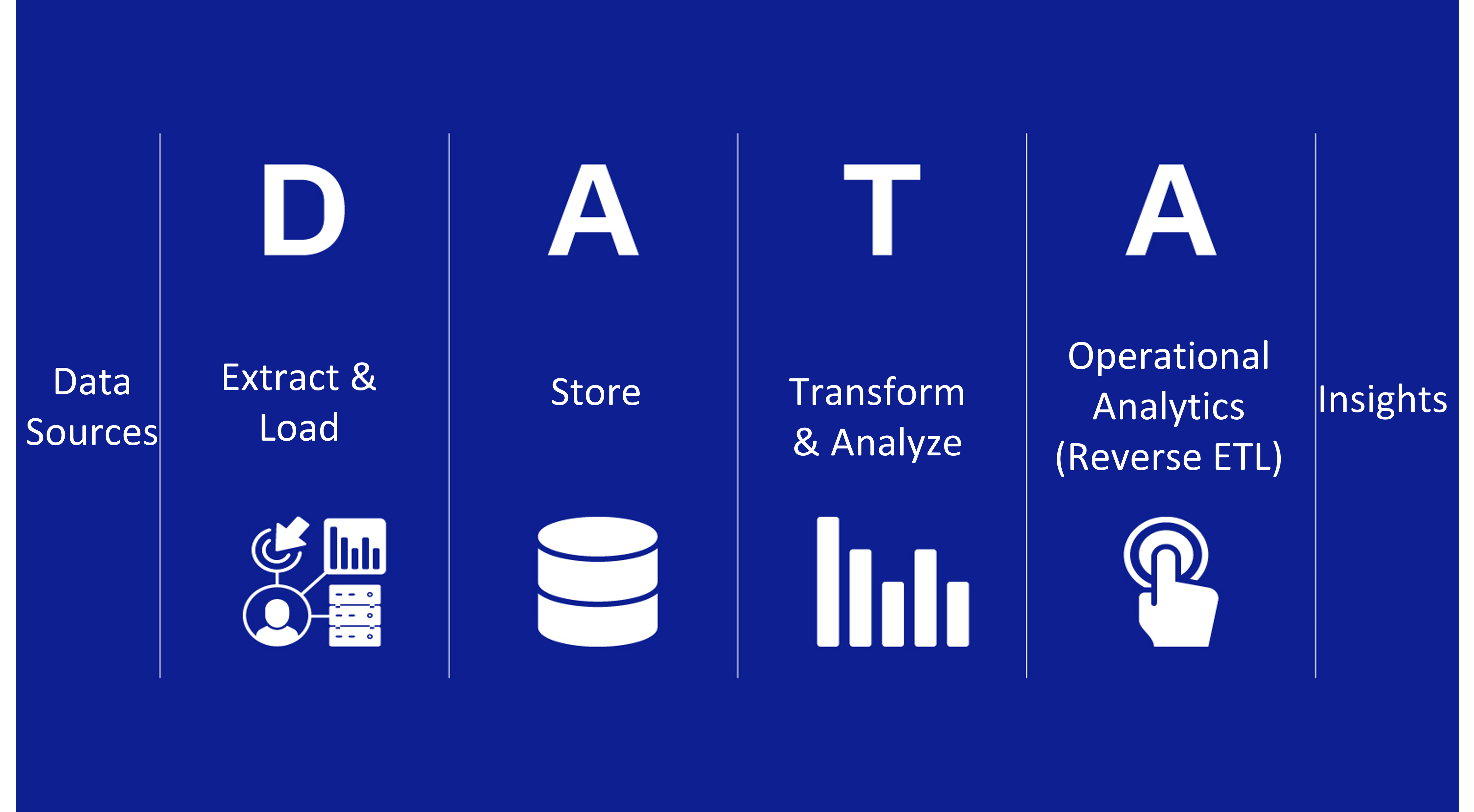
Operational Analytics - Who is it for?
Operational Analytics or Reverse ETL is not only limited to a few teams or departments but is actually a new way of functioning for the entire organization. The one where everyone has visibility of the same high quality data and insights to make highly informed decisions. If you have previously seen great ideas come out of data driven analysis only remaining confined to conference rooms and PowerPoint decks, then the results this new layer can bring will truly astonish you. When everyone in your organization has real time visibility to data they need and in the tools they need it in, it can truly revolutionize the working of the entire company. This will not only help decision makers but also create a feedback loop where you are constantly feeding quality data, using it for daily functioning and improving it before it is ingested back in again. Your teams will be able to iterate on ideas continuously and also be able to measure the impact. This virtuous cycle will improve your data quality and analytical models iteratively. All the business functions working in synchronicity with a single reliable data repository will provide a true competitive advantage. Now that we know the tremendous impact it can have, let’s see some of the use cases and ways different teams can make use of this.
Some use cases for Operational Analytics
While Operational Analytics can have virtually unlimited number of use cases, most companies start using Reverse ETL tools for automation of some routine high impact tasks like managing audience segments in various advertising platforms, product analytics and for making up to date, clean data available to the sales and marketing teams available in their native tools and platforms.
Sales Teams
By making high quality data about the customer behavior patterns and product usage available to the sales teams, Operational Analytics can ensure they can reach the right customers, with the right message at the right time in their journey with the product and the company. While this can be invaluable in Product Led Growth (PLG) companies, it is equally important in sales led organizations where crucial data points like lead scores and customer engagement metrics, data from support platforms if made available to the sales teams can revolutionize their functioning.
Data Teams
The most obvious and important advantage of adding Operational Analytics to the stack for data teams is the reduction in the work most of them dread doing - building custom integrations and fire fighting to just keep them working. They would ideally like to partner in strategic decision making by focussing on deriving insights from data. By making fresher, better quality data available to their “data consumers” they can not only benefit the company but also do so while retaining complete control over the data pipelines.
Marketing Teams
In most organizations Marketers are tired of requesting, chasing after and waiting forever for data from the data teams and always wishing they knew SQL and were able to just get to the data they need from the data warehouse. Guess what? With Operational Analytics digital marketing teams can. No longer do they have to wrestle with CSV files or unwieldy data dumps in Excel. They can now run hyper segmented campaigns and rapidly run experiments with different audiences through actionable user data. All of this can make running data driven, high performing and extremely high ROI marketing campaigns a reality.
Product Teams
Most product teams are already using platforms like Amplitude, Mixpanel, Moengage etc for product analytics. These platforms have a lot of data about user behavior, but they do not have a lot of contextual information which is organization specific. Using Operational Analytics or Reverse ETL tools, product teams can further enrich the data in these analytics platforms with specific contextual data to get much more granular visibility into user behavior, helping them segment their customers better and make more informed product strategy decisions.
Customer Success Teams
While for most customer focused companies, CS has been a very important function, it is even more important in today's day and age where there is intense competition for customer attention and often the deciding factor between a customer delight and churning is the support they get. In the case of PLG companies, this not only has an effect on renewals and churn, but is also directly responsible for revenue expansion. It is therefore important that the CS teams and sales teams are completely aligned and have clear visibility into all data related to the customer. This is made possible by Operational Analytics where using Reverse ETL tools, data is kept synchronized between the sales and CS tools and the data warehouse keeping everyone on the same page.
Operational Analytics - Prerequisites & Challenges in implementation
In order for an organization to start thinking about Operational Analytics implementation , they first need to have the other components of the data stack aligned. Which means they need to have a data warehouse set up along with ETL pipelines set up following the best practices for the same, ensuring that the data being ingested is complete and of the highest quality. Using a best in class ETL or data integration platform like DataChannel can help an organization get to this stage within days instead of months it would take them otherwise. Once you have this set up, the next step is to start syncing data to the business tools. Again the choice is between building your own pipelines to move this data or to use a pre-built platform for Reverse ETL to do this heavy lifting for you. This is where choosing a platform like DataChannel for your ETL needs seems like an even wiser decision as it also provides the functionality of Reverse ETL within the same platform, saving you months of time and thousands of dollars. Considering that even without the technical challenges, it is still a big paradigm shift for the organization to work in this manner, it is recommended to take small steps and activate one business function or process at a time. Often simple steps like setting up slack alerts on top of your data warehouse to get notified of metrics or when certain trigger conditions are met is a great starting point to get your teams used to this.
How do I get started with Operational Analytics?
Our advice would be to start small by activating simple processes using a tool like DataChannel. Set up a call with our experts today to get a demo and learn more or sign up for free to test it out on your own.
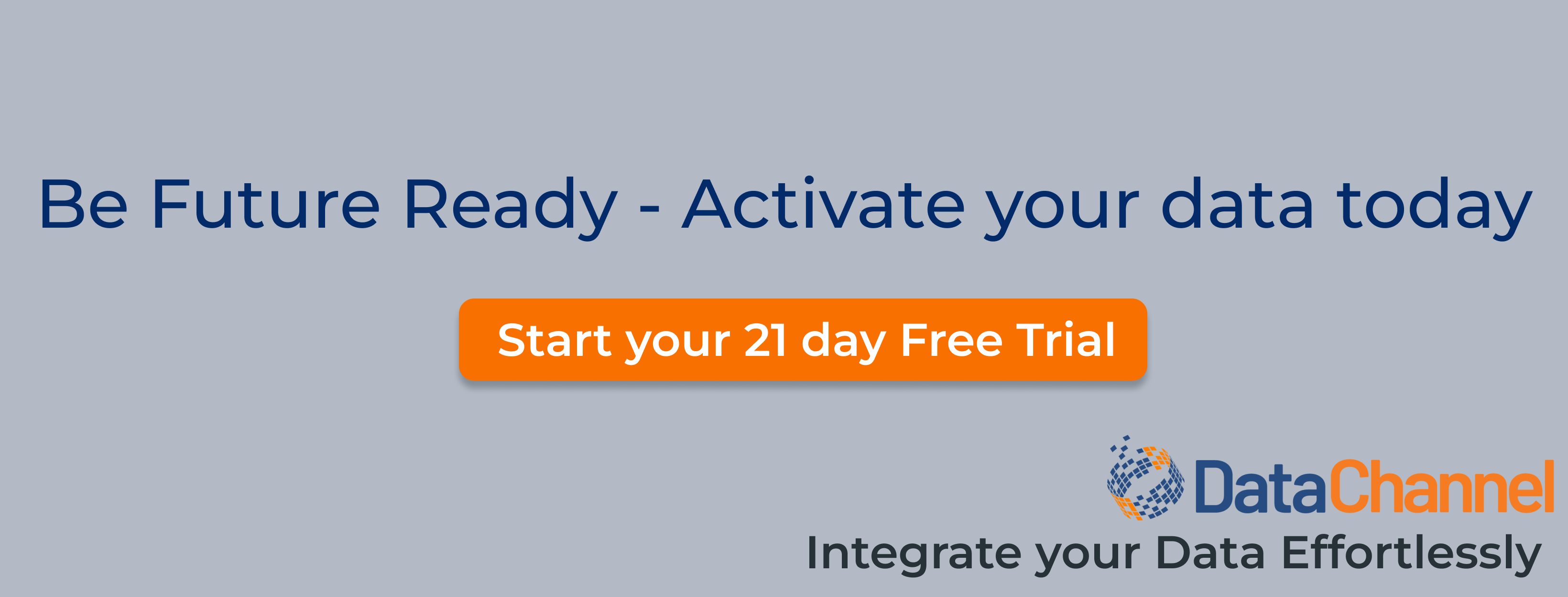