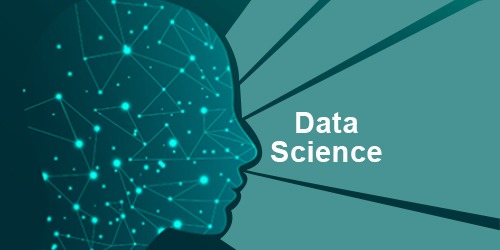
Is AutoML the Answer to the Data Science Skills Shortage?

In the data-driven business world, the demand for skilled data scientists has surpassed their availability. Skill shortages are restricting many organizations to make optimum utilization of the available business data and create models that can boost the pace and effectiveness of project completion. In the current situation, where the competition level is intense, every organization wants qualified workers having good technical skills, domain knowledge, and business expertise. But they need more qualified personnel to look out for technological solutions that can help them to make the best use of large volumes of data generated daily.
Here is when Automated Machine Learning makes a grand entry. The solution automates the process of applying machine learning to real-world problems. The AutoML involves a high degree of automation that allows even a non-expert to use machine learning models and techniques without having much technical knowledge. The technology has helped to meet the skill shortage by automating the task of data integration and model creation. With this technology on your end, your available data scientists get spared from the tedious job of data consolidation and shift their focus to other crucial tasks.
AutoML tools that can excellently automate the machine learning process:
- Auto Folio: It is an algorithm selection tool that assists developers in selecting the correct algorithm with accurate hyperparameters. It not only saves time but also makes use of the best algorithms to derive accurate results. To find out the best algorithm, Auto Folio chooses from a set of algorithms and reaches for the one that is most expected to solve a given problem efficiently.
- Amazon Alex: It is a deep learning technology that helps in building advanced chatbots in a less time-consuming manner. The chatbots built by it offer a brilliant user interface that can effectively respond to human conversations.
- Auto scklearn: The software has unlimited availability of machine learning algorithms. It helps data scientists to select the right algorithm with accurate hyperparameters that guarantees the best results. It offers out-of-the-box and result-driving machine learning techniques to scientists.
The AutoML tools enhance the workflow of analysts and data scientists by allowing them to test multiple algorithms and hyperparameters in no time. The right algorithms will help analysts to navigate the best route to solve problems for favorable results.

Is AutoML the answer to meet the skill shortage of data scientists?
The answer to the above question is no. Automated machine learning cannot automate all the data science tasks. It only helps data scientists to get relieved from the burden of performing repetitive and less demanding tasks and focus on those that are more challenging, require out-of-the-box solutions, and are harder to automate. Let us explain how-
On average, data scientists spend around 80% of their time consolidating, cleaning, formatting, and organizing large data sets. This leaves them only with 20% productive time to focus on solving other crucial data science problems. Even some organizations require data experts to get better insights into their business from the data sets they have. With automated machine learning, all the mentioned processes can be automated with zero human intervention. The software makes use of machine learning models with advanced systems so that the data scientist can move their focus to more critical issues.
The democratization of machine learning reduces the requirement of data scientists to a minimum extent. The data scientists have to follow a whole range of steps to build a machine learning model, like the collection of raw data, filtering the collected data, selecting and training an algorithm, and testing the functioning of the algorithm. And in case the algorithm fails the expectations, the data scientists have to repeat all the steps until they get the best algorithm. On the other hand, AutoML can create and test multiple algorithms in less time to deliver you the best one.
With automated ML, organizations can derive the same or even better results, and that too at a lesser cost and time. The datasets of different algorithms applications tested and recorded earlier by various data scientists provide a pre-designed data analysis structure to apply the right algorithms that bring positive outcomes.
But still, there are some objections to AutoML as data scientists feel that the solution cannot help with all the data science tasks. Its major purpose remains to relieve data scientists from tedious and repetitive tasks. The data-driven industrial segment will still be needing the support of skilled data experts to maintain the workflow of data science.
.png)