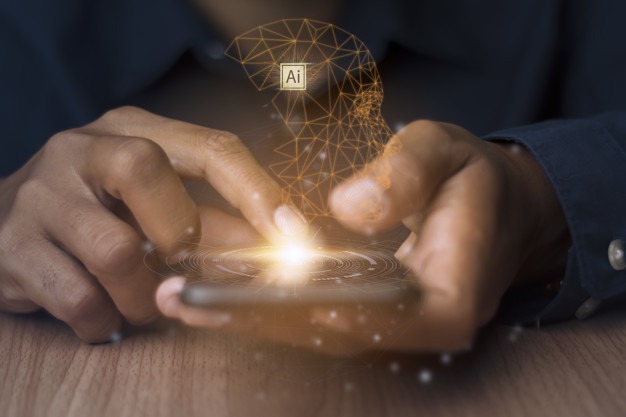
Automated Machine Learning Will Unlock Enterprise AI

The data-driven industry is in a tremendous need of data scientists to build models that will help them carry their organizational activities more efficiently. The big issue is that the number of trained data scientists in the industry is scarce, but what is becoming a bigger issue is that the models developed by these scientists are not efficient enough to make it to production. To make these models become operationalization, AutoML steps in.
Automated Machine Learning, or we can say AutoML, helps in meeting the scarcity of the data scientists by developing machine learning and deep learning models. With the AutoML system, you can provide the training data as input and get an optimized model as output. The software tests the model types and tunes their parameters in a way that it exactly fits the organizational needs. The AutoML helps the data scientists to move their attention from model creation to other core business processes to develop better business and market insights that will result in the growth and success of an organization.
The organizations that are about to start their AI journey require models that can efficiently be deployed in the business processes to create more value. Without an efficient model deploying capability, AutoML will be a software that creates a pile of undeployed models that never make into the business to automate the working of core business activities.

Learn more about ETL tools here
Another major benefit of using AutoML is that it can facilitate business intelligence and analytics by giving non-tech personnel access to ML technology within the tools they currently use. Now, businesses can easily perform data analysis (no matter how big and complex the data sets are) without the assistance of a database engineer. AutoML plays a significant role in the working life of data scientists as it helps them to divert their focus from the tedious task of model creation and testing to other critical activities that will shape the growth of the business in the dynamic market.
Model creation will be useless if it is not accurately deployed in the business processes. Every department of your company will be somewhere impacted if the model deployed is not working well. Let us take an example: as an HR of the company, you have created a model using AutoML and deployed the same to make decisions of career advancement and payroll increase of the employees based on their performance efficiency and time devoted to the organization. In case an employee gets a low pay increase and blames that the model deployed is biased, then the organization may have to prove that the deployment of the model was accurate and is working efficiently without any fault. To ensure transparency, generating a detailed paystub for each employee can be helpful. A comprehensive paystub not only outlines the breakdown of salary, bonuses, and deductions but also offers insights into how performance factors contributed to the pay decision. This level of detail can reassure employees that their compensation is fair and based on objective criteria.
The increasing adoption of AutoML is that it creates numerous “shadow AI” teams that create and deploy models without having the overall organizational visibility, accountability, and control. The inefficiency of these models to take into account every aspect of an organization, the different departments started using Cloud and SaaS apps that involve more cost and the risk of compromising with sensitive data.
Building centralized AI deployments has become necessary to take into account every aspect of each department to make decisions that will bring outcomes to an organization. It has become crucial to implement tools in business processes that will provide wider visibility, accountability, and control over the entire business activities.
AutoML helps in creating models and also tests them on the basis of different parameters, but this not guarantees their successful deployment in the core business processes. The efficiency of the models can only be measured when they are under operation. The topic will always be in debate for the next several years until we create a tool that will ensure 100% successful operationality of models in core business activities. With great operationality of these models, the data scientist can move on to other manual work to develop accurate insights for ensuring the success of the business in the short as well as long run.
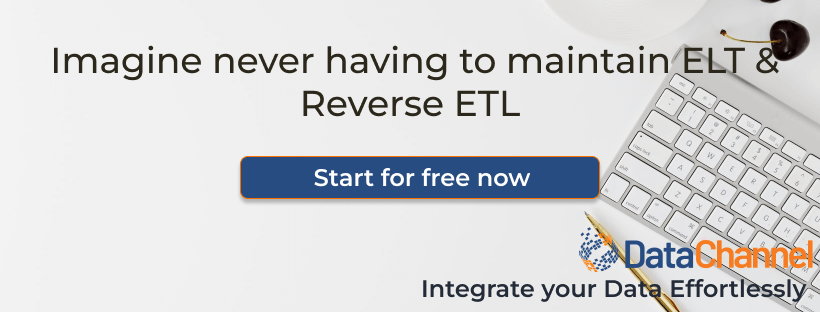
Conclusion
AutoML has a great way ahead in unlocking enterprise AI. Deploying models that can handle all the tedious manual processes with accuracy and efficiency is what a data-driven industry is longing for. They can aid in managing the scarcity of data scientists in the industry where even a small business decision depends on the legitimacy of the data.